WRF Data Assimilation (WRFDA)
A flexible, state of the art atmospheric data assimilation system that is portable and efficient on available parallel computing platforms
Current Version 4.4
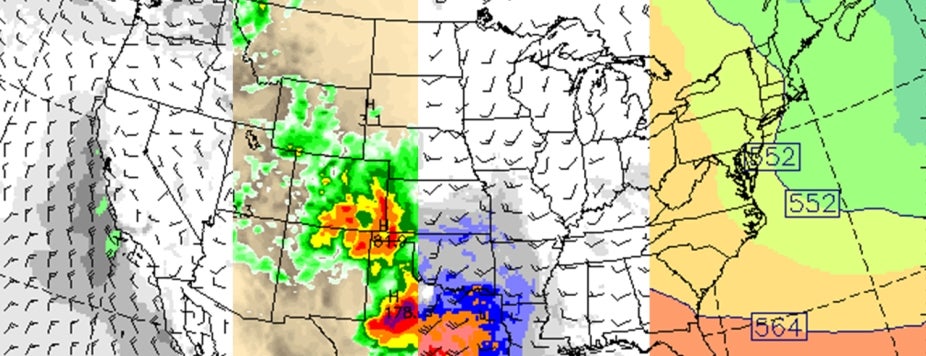
Downloads
The WRFDA system is in the public domain and is freely available for community use. It is designed to be a flexible, state-of-the-art atmospheric data assimilation system that is portable and efficient on available parallel computing platforms. WRFDA is suitable for use in a broad range of applications, across scales ranging from kilometers for regional and mesoscale modeling to thousands of kilometers for global scale modeling.
The Mesoscale and Microscale Meteorology (MMM) Laboratory of NCAR currently maintains and supports a subset of the overall WRF code (Version 4) that includes:
- WRF Software Framework (WSF)
- Advanced Research WRF (ARW) dynamic solver, including one-way, two-way nesting and moving nests, grid and observation nudging
- WRF Pre-Processing System (WPS)
- WRF Data Assimilation System (WRFDA) (found on this site)
- Numerous physics packages contributed by WRF partners and the research community
Techniques and Applications
The development of the radar data assimilation (DA) capability for WRFDA began in early 2000’s using the 3-dimensional data assimilation (3DVar) technique. In recent years, the capability has been expanded to the 4DVar system and enhancements have been made to produce improved convective forecasting, including new reflectivity operator, new momentum control variables, and cloud control variables. These enhancements have been included in the recent new release of WRFDA 3.7.1. In this presentation, an introduction of the radar data assimilation techniques in WRFDA and the recent enhancements will be provided for both the 3DVar and the 4DVar components. Examples from applications of the WRFDA radar DA system in different regions of the world for research as well as realtime operational forecast will be presented. Recent and future research activities aimed at improvements of multi-scale balance and background error statistics using hybrid approaches will also be discussed in the presentation.